AI for Social Issues
Members
Florence, Ebrima, Jiang, Komori, Huang, Nakamura, IZZUDDIN, Abdulmalik, He
Summary
The business applications of artificial intelligence (AI) have been all over the news. Industries from manufacturing to insurance are implementing ways to utilize artificial intelligence, sometimes alongside other emerging technology like machine learning. In addition to businesses, AI can have a significant impact on real-world social challenges and the potential to bring valuable solutions to various societal issues. Some projects in our lab weigh in on how innovative applications of AI can be used to combat some of the seemingly unsolvable social crises facing the world today.
One of these projects is to explore how intelligent systems can be responsibly applied to safeguard society against digital threats. One of our ongoing projects focuses on the development of an AI-based deepfake detection system to support the verification of video evidence in criminal justice.Deepfakes, created using deep learning models such as Generative Adversarial Networks (GANs), can produce highly realistic and convincing video forgeries. These manipulated videos are increasingly being used for malicious purposes, including misinformation campaigns, reputational harm, and tampering with legal evidence. In this research, we are developing a robust detection pipeline that can analyze videos frame-by-frame—even under poor-quality conditions like those found in surveillance footage—to determine their authenticity with high confidence.The system works by applying deepfake detection models and explainable AI techniques to evaluate each frame’s likelihood of being manipulated. As illustrated in the figure, the proposed system processes input video clips and produces frame-level fake probabilities. For example, the system correctly identifies a forged video as 96.87% fake by the 5-second mark, clearly surpassing the confidence threshold. Additionally, heatmaps are generated to highlight the manipulated regions in each frame, providing visual explanations to support forensic analysis.This research aims to empower law enforcement and forensic experts with an interpretable and transparent tool that can assist in the reliable screening of visual evidence, ensuring that only authentic video materials are used in criminal investigations and judicial proceedings
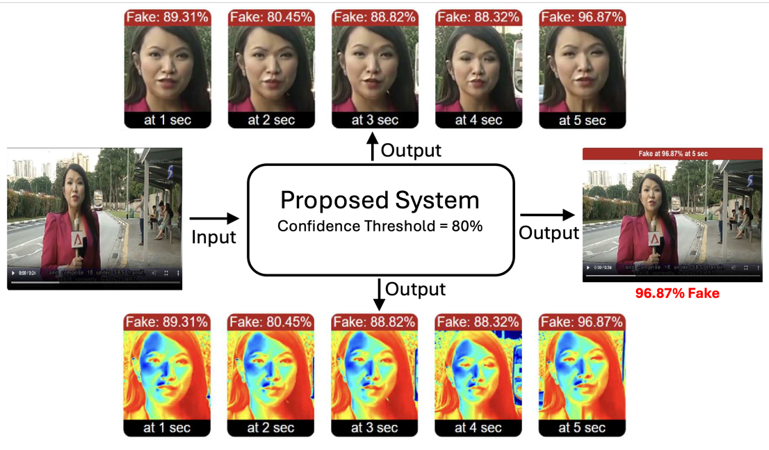
Another one consists of utilizing Natural Language Processing techniques of Question and Answering using Bidirectional Encoder Representations from Transformers to detect delay risk sources from unstructured project documents. Delay risk in construction projects is one global phenomenon experienced by the public sector causing socio-economic challenges. The main objective of this research is to eradicate this challenge by creating an Artificial Intelligence (AI) system that can predict and analyze this risk before its occurrence.
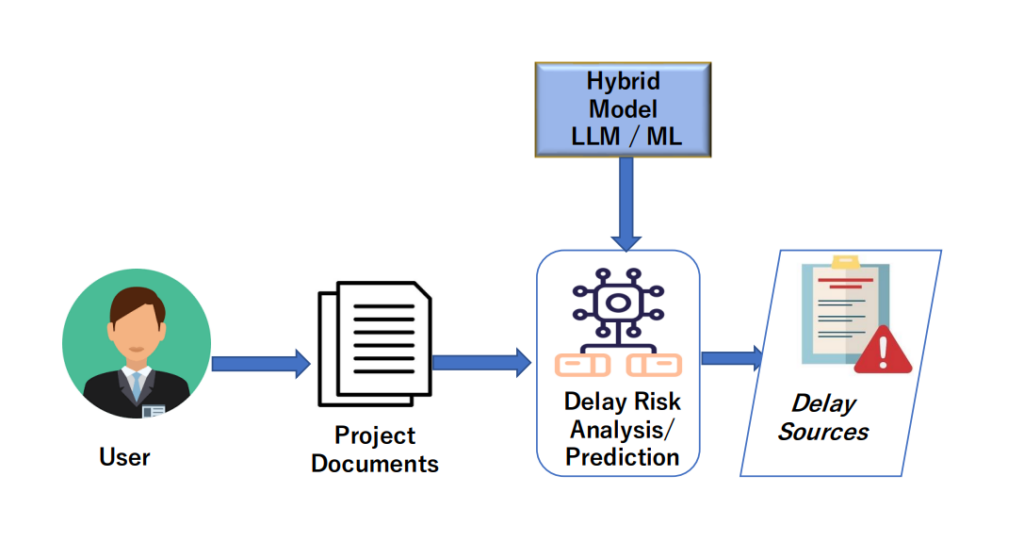
Another one consists of aiming to help small apparel stores create POP (Point of Purchase), we attempted to build an AI system that would help them concretize their intended customers and come up with ideas for how to express them. Utilizing a large-scale language model (LLM), the system helps even non-experts create appealing POPs by clarifying customer profiles through question-answering, improving expressions through stylistic changes and rephrasing, and automatically evaluating from multiple perspectives. Evaluation experiments showed that the appeal of POPs can be greatly improved by using this system.
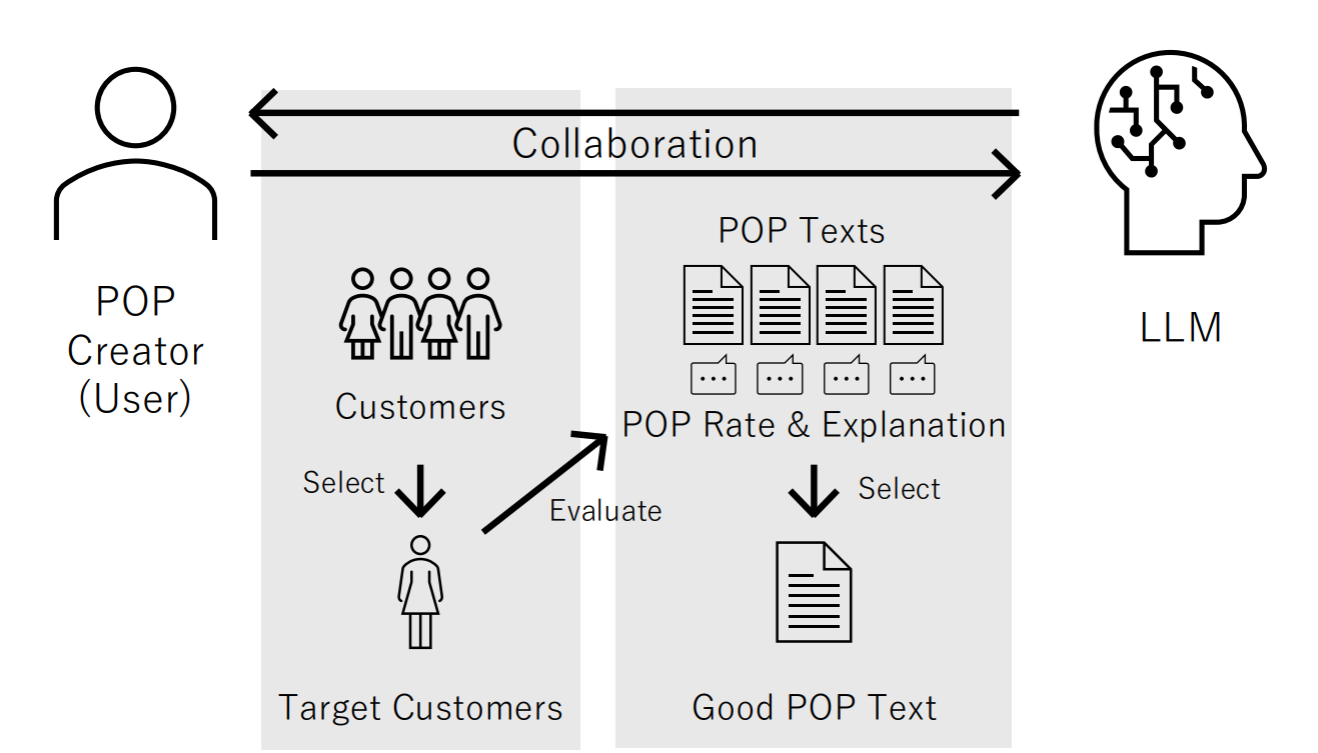
The final research is to develop and fine-tune a multi-model system designed to handle various aspects of Japanese text processing. This research integrates different models specialized for specific tasks to provide a comprehensive solution for the generation and translation of various Japanese texts. By leveraging the strengths of these specialized models, the system aims to achieve high quality Japanese language and contextual relevance in its output.
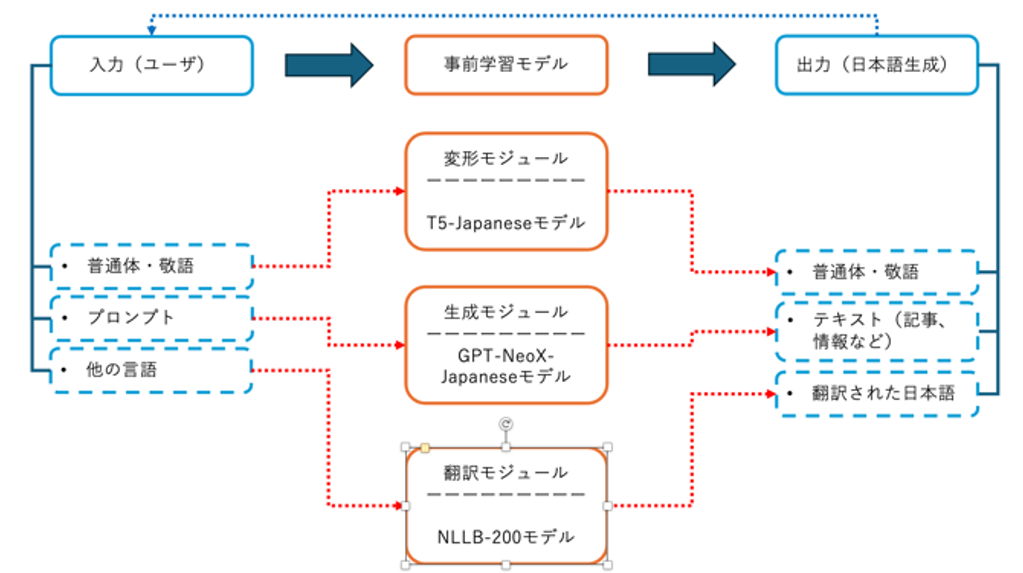