AI for Education
Members
Ezoa, Suzuki, Kato
Summary
Artificial Intelligence (AI) has the potential to address some of the biggest challenges in education today, innovate teaching and learning practices. AI has already been applied to education primarily in some tools that help develop skills and testing systems. As AI educational solutions continue to mature, the hope is that AI can help fill needs gaps in learning and teaching and allow schools and teachers to do more than ever before. That is why, some projects in our lab focuses on creating innovative AI approaches to solving problems schools, students and teachers are facing everyday.
One of the research projects in this category is the creation of video lessons for online classes. In particular, creating video lessons that involve pointing actions, like offline classes, is time-consuming. Therefore, we aim to create a system that can generate video lessons with pointing actions from slides and presentation manuscripts.
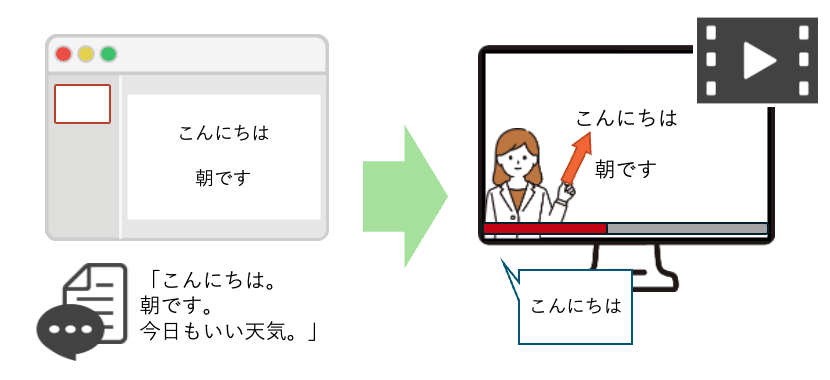
In addition, effective instruction in private tutoring schools requires the prompt presentation of teaching materials in response to the needs (questions, etc.) of each student. In this research, we have developed an agent that can autonomously present appropriate images from the dialogue between instructors and students, which does not include explicit search requests.
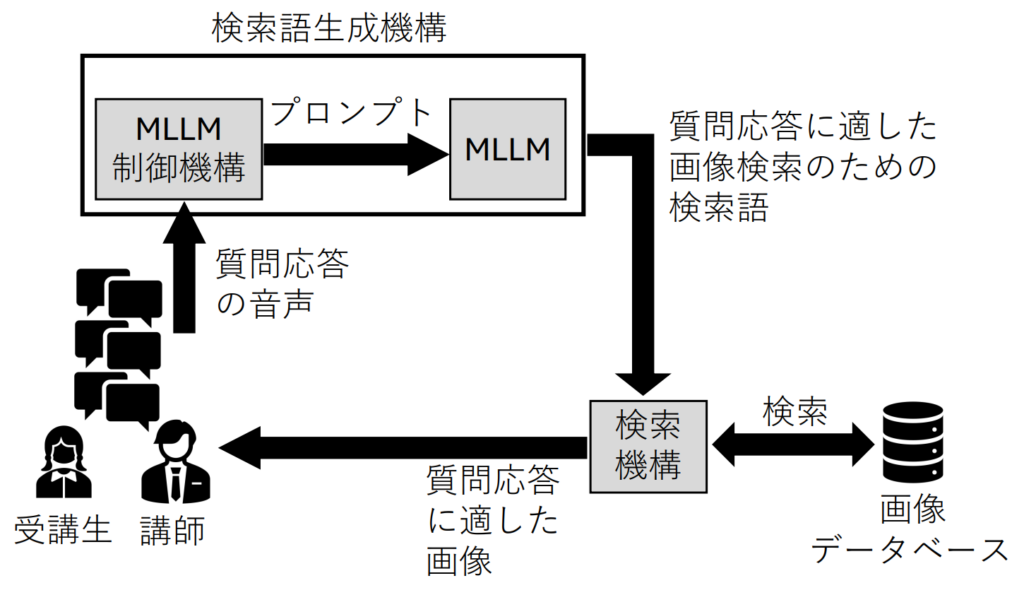
The COVID-19 period accelerated the transition from offline to online education. Despite the advantages of this new mode of learning, the automatic evaluation of students remains a challenge. This research aims to introduce a new coefficient for evaluating student confidence during lectures by assessing their abilities and determining whether the lecture content is perceived as challenging.
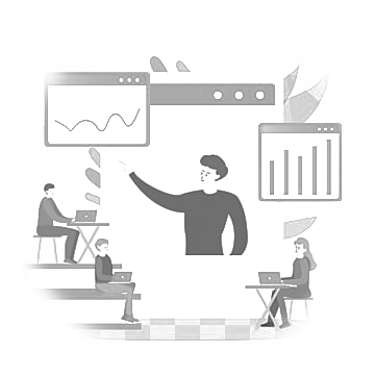